More recent conceptualizations of resilience highlight it’s multifaceted and dynamic nature. Thus, resilience can be viewed as a process (e.g., reflected in the trajectories of adverse psychological symptoms and subjective well-being measured over specific time windows during illness). These trajectories are expected to reveal patterns of stability (e.g., consistently high levels or consistently low levels of anxiety over time), lessening, or worsening of symptoms during the crucial months following diagnosis and during cancer treatments. Resilience as a process has been investigated in previous smaller-scale studies with solid theoretical models regarding trajectories. As more measurement waves (follow-up assessments) become available from the BOUNCE study, advanced statistical and computational models can be applied to explore the complex interplay of treatments side effects, life events, emotional, behavioral, and cognitive processes over time. Results of such models will be part of the next BOUNCE newsletter.
Current computational modeling of BOUNCE data, using supervised learning algorithms, has been applied to the definition of resilience as outcome. Within this framework we have addressed two main clinical objectives:
- To achieve optimal prediction of one-year patient outcomes (in terms of symptoms of good mental health and good overall quality of life or subjective sense of well-being) using all available information aggregated over the first 3 months post diagnosis, and
- To identify potentially modifiable patient characteristics that determine one-year mental health and/or quality of life outcomes. The latter models did not include mental health and/or quality of life measurements obtained during the first three months post-diagnosis in order to enhance model sensitivity towards more clinically useful psychological characteristics (such as optimism and emotional self-regulation strategies) and behaviors (i.e., coping strategies such as exercise).
We have tested this machine learning framework on the fully annotated BOUNCE dataset that became available as of May 2021. This dataset includes socio-demographic, lifestyle, medical and self-reported psychological characteristics of women recently diagnosed with breast cancer. Currently 12-month follow up data are available for 532 women aged 40-70 years.
The analysis pipeline employed to address these objectives entailed feature selection, model training, and model testing. Feature selection was conducted using a transformer built on Random Forest (RF) estimator which assigns weights to the most important features and ranks them according to their relative importance. The feature selection scheme was encapsulated with the classification algorithms into the ML-based pipeline in order to select only the relevant features to train the selected classifiers.
We took special care to avoid overfitting, which often results when model training and testing takes place on partially overlapping subsets of cases. To address this rather common problem in machine learning applications in clinical research we adopted a cross-validation scheme with holdout data for final evaluation. In this manner, model testing was always performed on cases that were not considered during the training phase and, consequently, did not influence the feature selection process. This procedure helps to increase the generalizability of predictions and the accuracy of the final models when applied to new cases in the future. Specifically, a 3-fold Split for Hyper-Parameters tuning and Model Evaluation (train, validation and test sets) were applied after splitting the dataset into training and test sets. Α grid search procedure with an inner 5-fold cv was applied on the validation set for adjusting parameters and for selecting the best parameters of the trained models. Figure 1 illustrates the supervised learning analysis pipeline. Tree-based classifiers were applied within the ensemble methodology, such as Random Forest (RF), Decision Trees (DTs) and Gradient Boosting Machines (GBM) estimators.
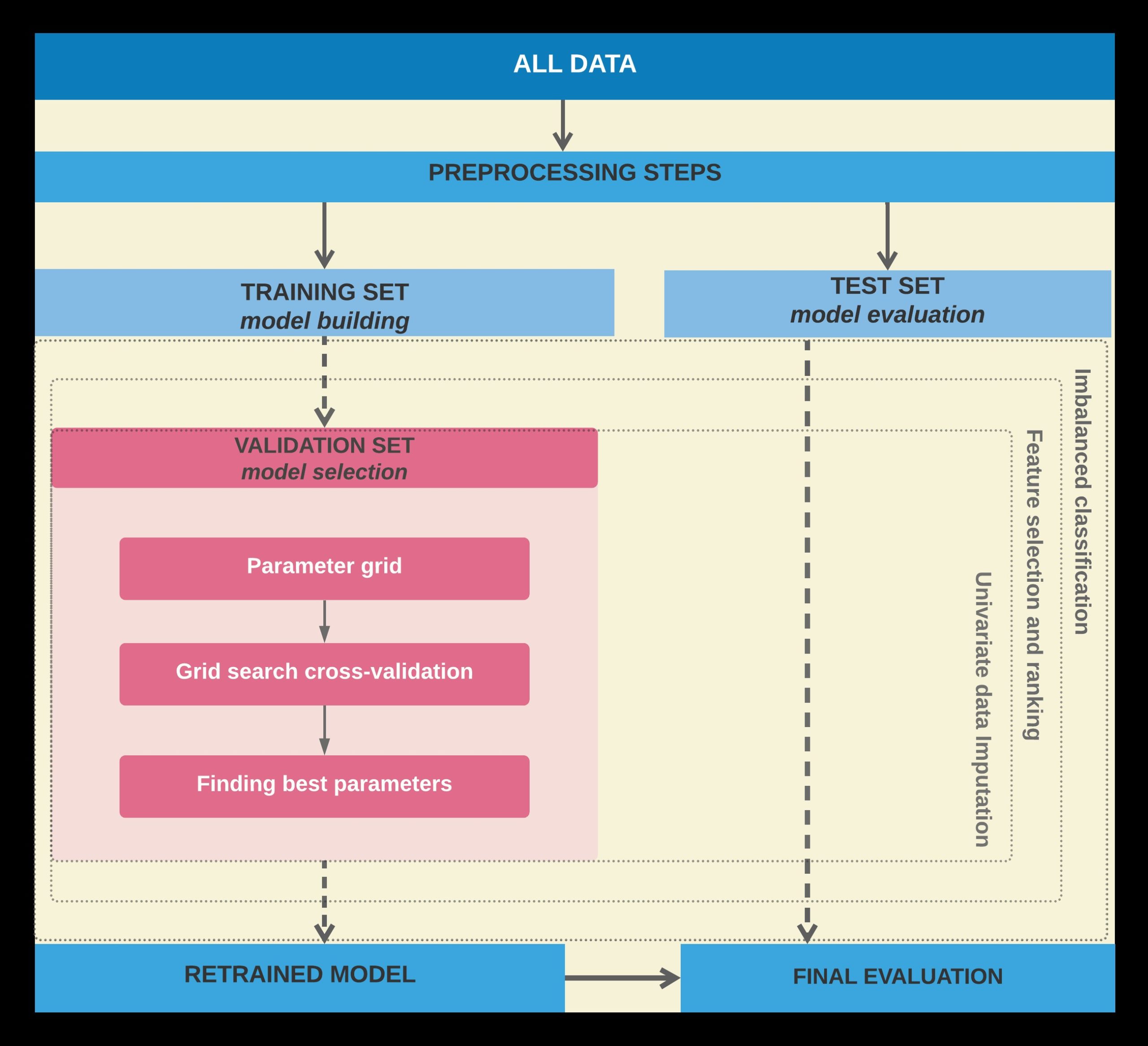
Figure 1. The data division scheme of the proposed supervised learning pipeline for training, testing and validation tests.
Initially, we assessed model accuracy for predicting who will show good resilience at one year post-diagnosis (in terms of anxiety and depression symptoms or overall well-being). All available medical, psychological, socio-demographic and lifestyle measurements, obtained immediately after diagnosis and shortly after (at the 3 months follow up), served as predictors in these models.
Predictors included overall mental health status and global QoL registered early during the course of the illness.
Table I. presents the classification performance of the two prediction models, revealing a fair balance between specificity and sensitivity metrics.
The shape of the Receiver Operating Characteristic Curve below supports this notion.
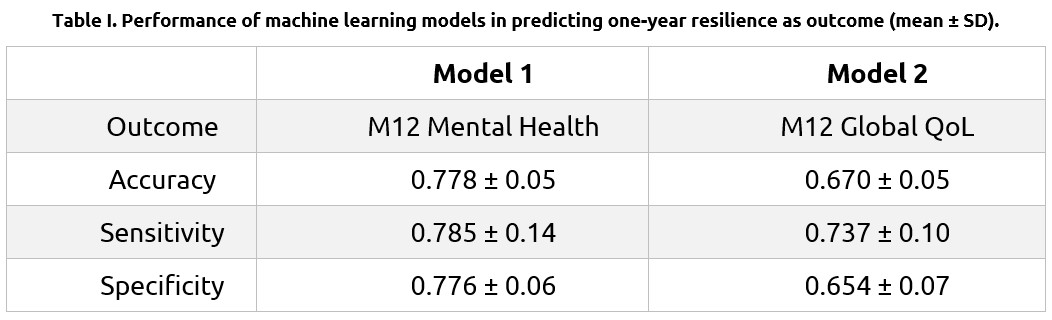
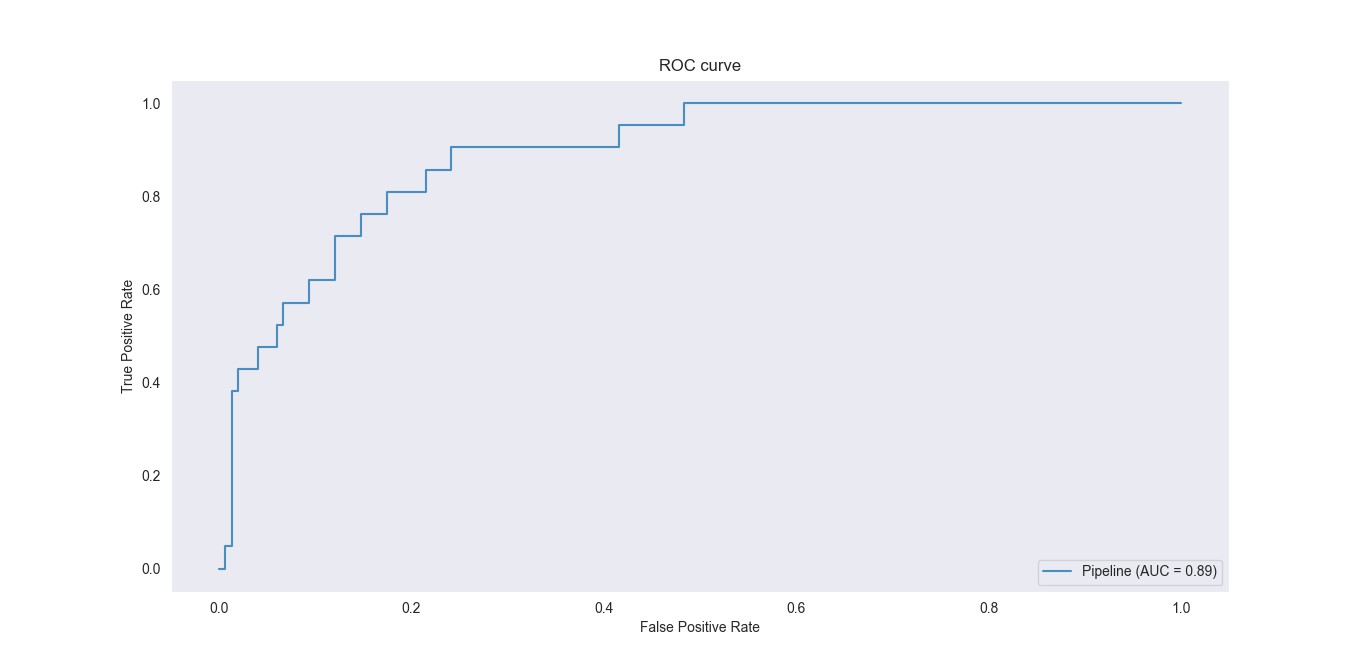
Overall, prediction of significant anxiety and/or depression symptoms was achieved in approximately 79% of cases (Model 1). Moreover, the model identified patients who develop such symptoms with approximately 78% certainty. Corresponding performance in predicting poor one-year well-being was somewhat lower (74% and 65%, respectively; Model 2).
In supplementary analyses, we sought to identify potentially modifiable behavioral and psychological characteristics that could inform clinicians regarding the needs of individual patients at the early phases of illness in order to maximize psychological resilience at later stages.
Model results related to the overall quality of life are presented here as one important instance of resilience-as-outcome. In view of the strong association between early and subsequent scores on the same self-report scales, scores on subjective quality of life obtained during the first 3 months of the course of the illness were excluded from the set of potential predictors of these variables as outcomes.
As shown in Table II, important predictors of 12-month quality of life included variables measured shortly after diagnosis, as well as variables, recorded three months into the course of the illness. As expected, overall emotional state (negative affect) and the experience of physical side effects (illness- or treatment-related) during this period featured strongly among predictors. There were however additional variables corresponding to psychological characteristics that can become the focus of systematic psychological interventions, such as ways of managing one’s negative emotions in response to stressful events, and ways of thinking about the illness and what it represents in one’s life. Lack of adaptive coping strategies is another characteristic that can be improved through intervention.
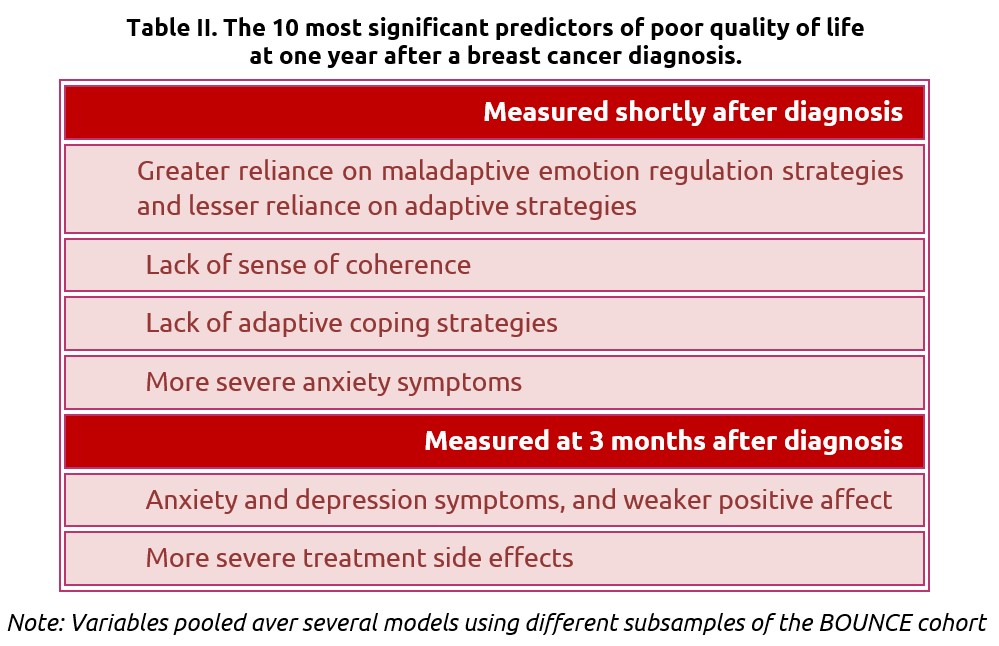
Finally, the generalizability of the prediction models was assessed across the four clinical sites that contributed to the BOUNCE prospective study. In these analyses, feature selection and model training were conducted using data from three clinical sites, and the developed model was tested on unseen data from the fourth clinical site. Results revealed very similar classification performance across the four learning schemes. Importantly, predictor variables were largely overlapping across sites, despite notable cultural and sociodemographic differences across the four subcohorts. These results inspire optimism regarding the successful transfer of our prediction models to other clinical settings across Europe.
These findings are in accordance with the major psychological theories about adaptation to severe illness, including breast cancer, such as the Common Sense Model (Leventhal, Phillips, & Burns, 2016) or the Transactional Stress Model (Lazarus & Folkman, 1987). According to these models, adaptation to a severe health crisis is a complex process that is determined by (a) a variety of personal (and interpersonal) resources, which may buffer the negative impact of the situation and facilitate adaptation; (b) cognitive-emotional processes (e.g., representations/perceptions of self and the condition) that guide behavior (e.g., coping behaviors); (c) contextual and specific stressor-related factors that may impact adaptation (directly or indirectly).
Indeed, the variables that emerged as significant predictors of one-year mental health and quality of life (resilience-as-outcome) correspond to these “clusters” of factors. For example, sense of coherence, self-efficacy to cope with cancer, or optimism are key personal resources that refer to a sense of control. Emotion regulation and illness representations (e.g., representations of illness consequences) are forms of cognitive and emotional processes that typically guide emotions and actions/behaviors (e.g., anxious preoccupation). The experience of side effects or the previous levels of well-being are parts of the broader context that defines patients’ experience (e.g., Hagger et al., 2017). In addition, the findings pinpoint those early factors (coming from a large array of sociopsychological, medical, and lifestyle variables) that are significant predictors of the final outcomes and, in this way, will guide our efforts to develop the clinical recommendations which may guide health professionals’ and patients’ informed decision making in order to achieve better adaptation and reinforce patient resilience.
Finally, it is worth noting that, besides side-effects (as perceived by the patient), the medical factors did not emerge as significant predictors of the M12 overall mental health and global quality of life. The most possible explanation might be that these factors exert their influence on mental health and quality of life indirectly; that is, through the cognitive-emotional, behavioral, and situation-specific variables described above. Future analyses will examine the potential indirect impact of medical factors on the outcomes.
References
- Leventhal H, Phillips LA, Burns The Common-Sense Model of Self-Regulation (CSM): a dynamic framework for understanding illness self-management. J Behav Med. 2016; 39:935-946.
- Lazarus RS, Folkman Transactional theory and research on emotions and coping. European Journal of Personality. 1987;1:141-169.
- Hagger MS, Koch S, Chatzisarantis NLD, Orbell S. The common sense model of self-regulation: Meta-analysis and test of a process model. Psychol Bull. 2017; 143:1117-1154.
By Konstantina Kourou PhD, Unit of Medical Technology and Intelligence Information Systems, Institute of Molecular Biology & Biotechnology (IMBB), Foundation for Research & Technology – Hellas (FORTH), Ioannina, Greece and Giorgos Manikis PhD, Computational Bio-Medicine Laboratory, Institute of Computer Science, Foundation for Research and Technology-Hellas, Heraklion, Greece
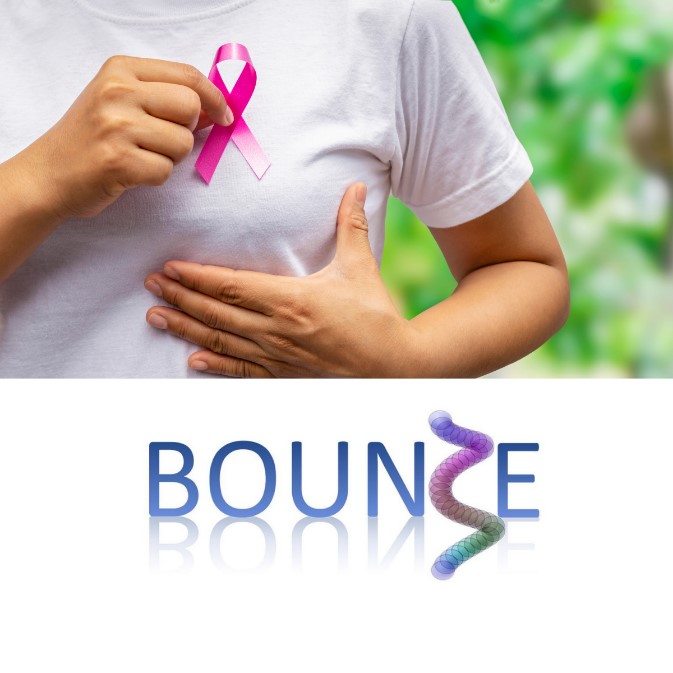
Medical doctors and mental health professionals alike have long recognized that many patients show better recovery and capacity to bounce back following similar, serious illnesses than others. For some time, it was believed that psychosocial resilience is a relatively stable characteristic trait on which persons differ, in the same manner that people vary from each other on personality characteristics. In this context, self-rated psychological characteristics can serve as one of several indices of a patient’s trait resilience (i.e., their perceived general ability to effectively deal with adversity, such as illness, or withstand its impact and consequences).